But before describing what we found, we need to look at what constitutes chocolate ... and in particular, what is meant by "cocoa solids".
What Is Chocolate?
"Chocolate is made from the kernels of fermented and roasted cacao beans. The kernels are ground to form a pasty fluid chocolate liquor ... pressed to reduce the cocoa butter content and then pulverized to make cocoa powder, or mixed with sugar and additional cocoa butter to make sweet (eating) chocolate."
https://www.britannica.com/topic/chocolate

While cocoa butter is a natural part of the cocoa liquor (also known as cocoa mass), chocolate makers often blend additional cocoa butter with the cocoa mass to adjust the flavour and texture of their chocolate. Together these two components - the cocoa mass and any additional butter - form the 'cocoa solids' usually listed on the label.
70% cocoa mass (with or without additional cocoa butter), and 30% sugar, is a common ratio for dark chocolate. Pure cocoa mass tends to have a bitter taste, and the added sugar makes for a more enjoyable taste experience for most people.
NIR Absorbance 'Fingerprints' for Chocolate
Just as milk chocolate appears lighter in colour compared to dark chocolate when we look with our eyes, when we scan chocolate with NIR spectrometers we can see similar differences iin the way that chocolate absorbs light in the near-infrared.
Using an experimental NIR spectrometer developed by ams-Osram to demonstrate the potential for lab-on-a-chip applications, we found that there are clear differences in the near infrared absorbance of chocolate just beyond what the human eye can see.

We found a similar differentiation by % cocoa solids with Sagitto's miniature hand-held NIR spectrometer, which operates at higher wavelengths. Here (for example) are the absorbance spectra for Lindt Excellence chocolate bars.

If we process the above Lindt chocolate spectra using a mathematical technique called Linear Discriminant Analysis ('LDA'), and plot the analysis in 2 dimensions, we can see the different chocolate bars clearly separated - with the 30% cocoa solids bar furthest from the 95% cocoa solids.

The same pattern is revealed if we apply Linear Discriminant Analysis to a range of chocolates from another manufacturer, Whittakers. Their 'White' chocolate (for which cocoa butter makes up the entire 28% cocoa solids) on the far left and "92% Ghana Intense" on the far right.

Single-Origin Chocolates
It is not surprising that we should be able to use near-infrared spectroscopy to readily distinguish between chocolates with different percentages of cocoa solids. After all, this is something that we can do to some extent with our own eyes. So what about chocolate bars which all have the same proportion of cocoa solids and sugar and which differ only in the origin of the cacao beans?
Foundry Chocolate produces an amazing range of single-origin chocolates, with no added cacao butter, lecithin or vanilla. When we apply Linear Discriminant Analysis to nine different bars of Foundry 70% chocolate, each bar with the same % cocoa solids but made from cacao beans from a different geographical origin, we can see clear differences (and similarities) between chocolate from different origins.

Measuring Cocoa Solids With NIR Spectroscopy and Machine Learning
We next decided to see if we could use machine learning to predict the percentage cocoa solids in chocolate.
.png)
We know that the composition of 'cocoa solids' varies depending not only on the source of the beans and the method of their processing into chocolate, but also on whether extra cocoa butter and other compounds (such as milk products) have been added. So we were pleasantly surprised to find that we could build a reasonably accurate machine learning model that would predict the % cocoa solids just from a single NIR scan.
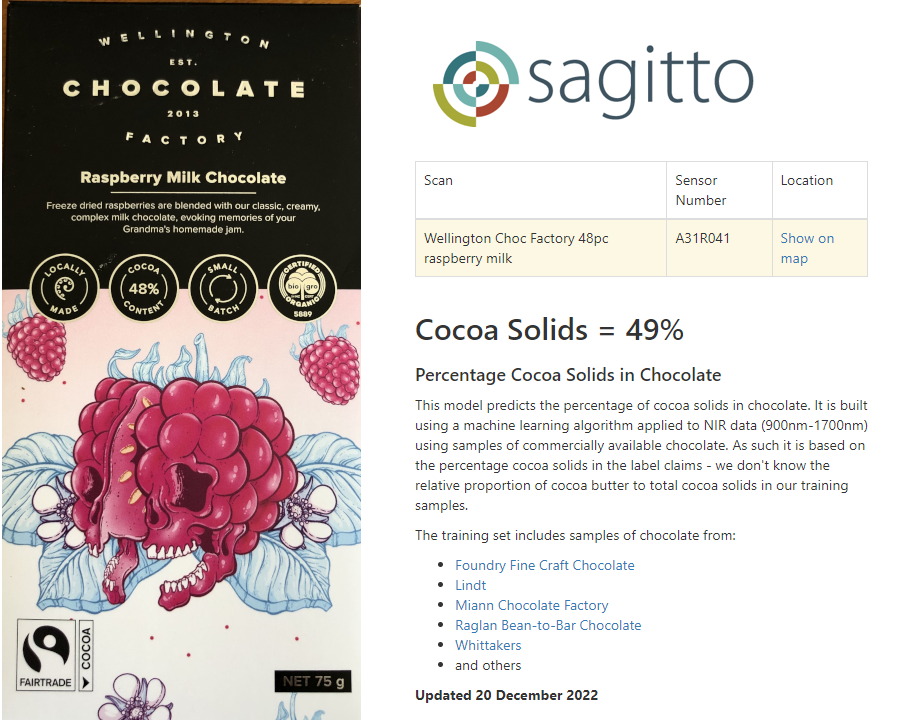
Measuring Chocolate Sensory Parameters With NIR Spectroscopy and Machine Learning
Studies such as this one conducted at the University of Melbourne's Sensory Laboratory have shown that accurate machine learning models can be built using near-infrared spectroscopy to predict not only physicochemical parameters in chocolate such as pH, Brix and viscosity, but also sensory parameters such as bitterness, saltiness, sourness, sweetness, and umami. The study concluded that:
"[machine learrning] models can be used as an alternative to consumer panels to determine the sensory properties of chocolate more accurately, with lower cost."
Conclusion
Our model for predicting coca solids was fun to create, but it is not very useful. After all, we can find out the % cocoa solids in a chocolate bar just by reading the packaging. However it does illustrate the discriminative power of NIR spectroscopy. We are confident that if we were to gather reference data for chocolate bars from a properly trained sensory panel then we would be able to develop accurate machine learning models to generate taste profiles for these chocolates.
We started this experiment out of curiosity, to see what we could learn and because we like eating chocolate! 70 bars of chocolate later, we've learned that there is considerable scope for combining machine learning with near-infrared spectroscopy to measure taste profiles - not just for chocolate but many other foods too!
Watch this space!
Footnote
The chocolate bars were all purchased in New Zealand, often from small speciality chocolate makers that pride themselves in sourcing beans direct from individual cacao farmers in the South Pacific, including:-
- Bennetto Natural Foods
- Foundry Chocolate
- Miann Chocolate Factory
- OCCHO (Otago Chocolate Company)
- Raglan Chocolate
- Wellington Chocolate Factory and
- Whittakers
Our reference data came from what was printed on the manufacturer's labels, or their websites. And for this experiment we used two NIR spectrometers to scan the chocolate bars to obtain their NIR absorbance fingerprints:-
- Sagitto's miniature NIR spectrometer, which measures in wavelength range of 900nm to 1700nm; and
- an even smaller NIR spectrometer supplied by ams-Osram, which measures between 750nm to 1050nm
A relevant scientific paper that we came across after writing this blog is the paper by Ingrid Alves Santos, Daniele Gomes Conceição, Marília Borges Viana, Grazielly de Jesus Silva, Leandro Soares Santos, Sibelli Passini Barbosa Ferrão called NIR and MIR spectroscopy for quick detection of the adulteration of cocoa content in chocolates, Food Chemistry, Volume 349, 2021, 129095, ISSN 0308-8146, https://doi.org/10.1016/j.foodchem.2021.129095.
Abstract: The Near (NIR) and Mid (MIR) Infrared Spectroscopy associated with chemometric techniques were used to determine the cocoa solids content in chocolates and detect possible adulterations. Five chocolate formulations (30% to 90%) were produced with different cocoa solids concentrations and 110 commercial samples from 10 different countries with varying concentrations of cocoa solids (30% to 88%) were acquired. All repetions of the produced and commercial chocolates were evaluated using NIR and MIR. Spectroscopic data were submitted to multivariate techniques of Principal Component Analysis (PCA) and Partial Least Squares Regression (PLS). For both spectroscopy techniques, the PCA of the 5 formulations formed 5 distinct groups regarding the cocoa solids and the commercial samples showed a behavior pattern similar to the produced samples. For PLS, the regression equations showed high predictive capacity, with correlation coefficients above 90 and RMSECV values of 0.70 and 1.22, for NIR and MIR, respectively. These models highlighted, approximately, 14% of the commercial samples as possible adulterated products.
Keywords: Authenticity; Fraud; Chemometry; Theobroma cacao