Building Predictive Models Using NIR Spectral Sensors and LEDs
As one of ams-Osram's Preferred Partners, Sagitto had the opportunity during the 2021 and 2022 Southern Hemisphere harvest periods to take NIR spectral measurements and reference data from fifteen different apple varieties - including Sunrise, Gravenstein, Royal Gala, Sweetango, and Envy.
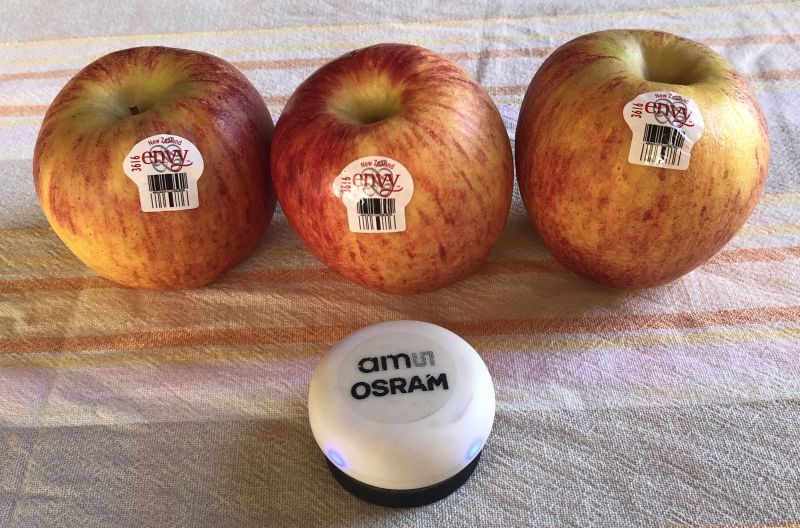
ams-Osram have developed a demonstration design for a miniature NIR spectrometer, using very small but powerful NIR LEDs as the illumination source and operating over the spectral range of 750nm to 1050nm. Just as we can often see differences in colour between different apple varieties with our own eyes, apple varieties differ in the way that they absorb light in the near infrared part of the spectrum too, as seen in this plot.
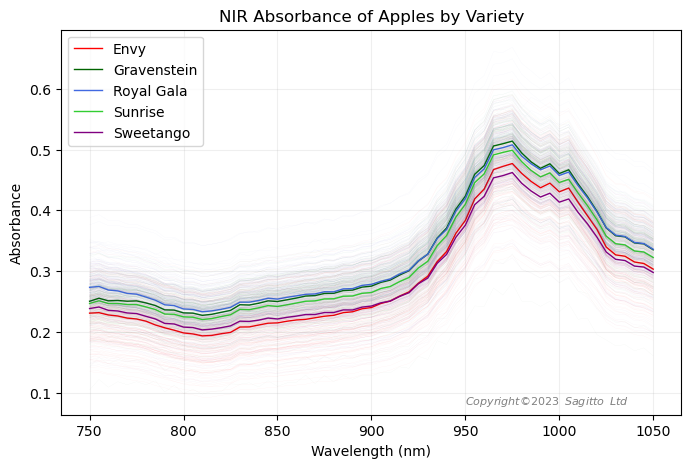
By combining the non-destructive NIR spectral data with destructive measurements of °Brix and Dry Matter from almost 1,000 apples, Sagitto was able to build very accurate predictive models for these two important apple quality parameters.


A Wide Range Of Apple Varieties
As the following histograms show, there are considerable differences in the profile of °Brix and Dry Matter between varieties. At one extreme is Sunrise, an early season apple that is naturally tart. At the other extreme, Envy apples are picked towards the end of the harvest season and have very high fruit sugars and dry matter.

A model that is built on only one variety might be accurate for that particular variety, but probably would not be accurate for other apples - especially those with very different °Brix and Dry Matter profiles.
Demonstrating Importance Of Variety
To demonstrate the importance of having representation from different apple varieties in our machine learning training dataset, we conducted an experiment: for each of five varieties - Royal Gala, Envy, Sunrise, Sweetango, and Gravenstein - and each of the two quality parameters, we built two regression models.
The first regression model included all five varieties in its training set, so that the variety had been 'previously seen' by the model. The second regression model was trained on all except the variety being investigated - what we've called the 'Never-Seen-Before' variety.
The results were as we expected. Regardless of whether we predicted soluble sugars (°Brix) or dry matter (DM%), for each of the five varieties the models that had previously seen examples of spectra from the target variety performed better than the models that had never seen apples from that variety.
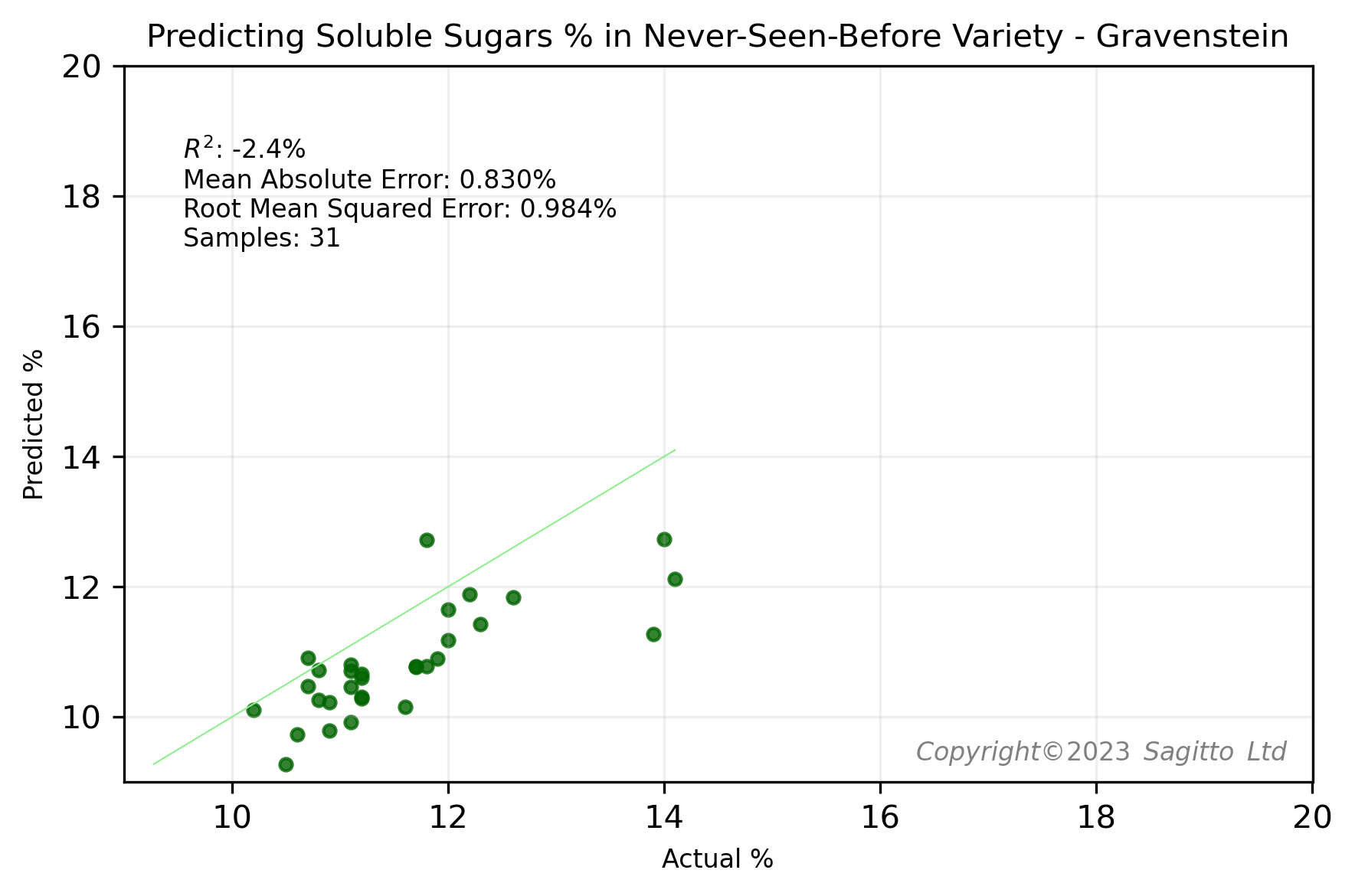

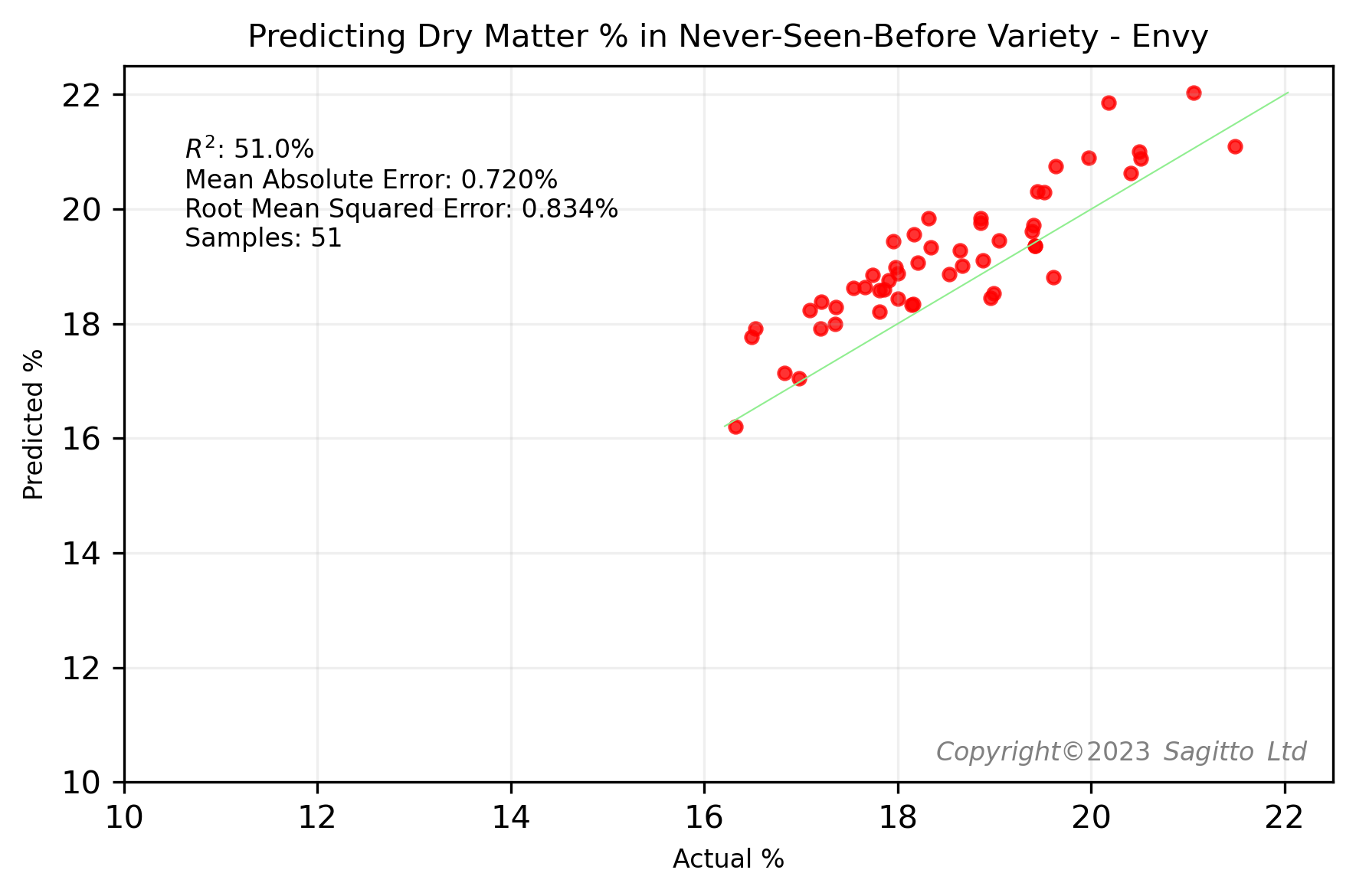

Do We Need Every Apple Variety?
We have demonstrated that ams-Osram's miniature NIR spectrometer can be used to accurately measure important quality parameters in apples, across a range of apple varieties, when those varieties are present in our training data - but there are thousands of different varieties of apple around the world.
We are not suggesting that a robust and accurate NIR model for apple quality parameters needs to include examples of all varieties - if we are only interested in testing one variety of apple, then we only require examples from that one specific variety. However, we have found that combining data from a range of varieties can lead to synergies: our accuracy for each variety can be greater than that of models built on a single variety's data alone.
Conclusion
Having a diverse and representative training set is crucial in machine learning because it allows the model to generalize better to new data. If the training set only contains a limited number of examples or is not representative of the population, the model may be 'overfitted' - that is, perform poorly on unseen data.
When developing NIR models to predict apple quality, it is important to carefully select the apple varieties in the training data.
Special thanks to the team at T&G Global of BayWa for supplying some amazing Envy apples for testing